In May 2023, we hosted a roundtable in partnership with artificial intelligence research lab, Google DeepMind, on how AI might enable personalised lifelong learning to drive better outcomes for people, places and planet.
The event was attended by policymakers, practitioners and learners working at the intersection of AI and education. In a three-part series of articles, we present the roundtable discussion insights to establish the current landscape of learning and AI, explore the challenges and opportunities for AI-enabled personalised learning, and speculate on its future trajectories. In this introductory article, we contrast the emerging landscape for our skills and how learning must adapt to emerging uses of AI. Ultimately, we assess what AI for personalised learning could look like in practice as a predicted future scenario.
Our education system needs to adapt
With the interconnected environmental, societal, geopolitical and economic polycrises humanity is currently facing, it is becoming clear that our human capacity to adapt, evolve, learn, unlearn and relearn will be critical over this coming era. As technological advances, ageing populations and climate change shape new economies, workforces will need to adapt to keep up and will be in need of dramatic upskilling and reskilling.
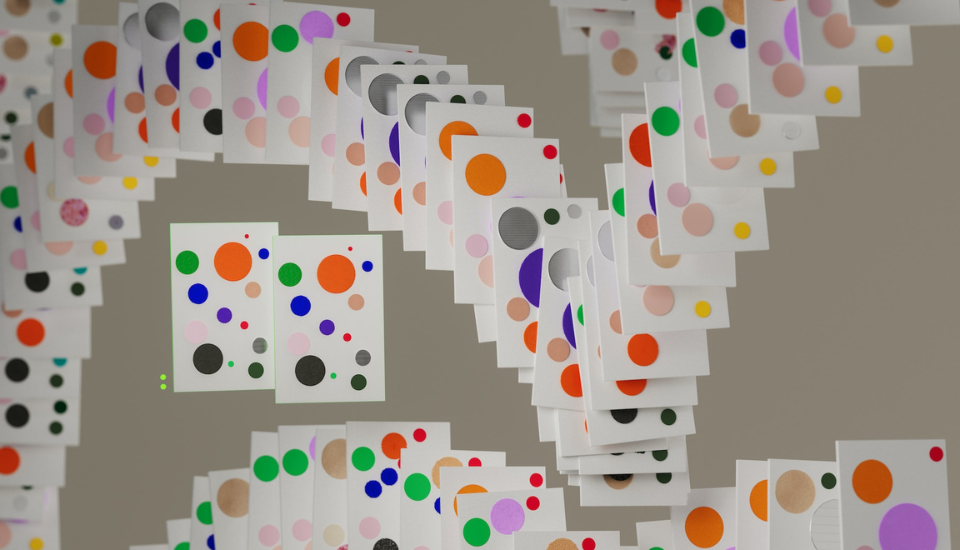
The capacity to learn – and continue learning throughout life – has never been more important as we prepare to rapidly adapt to continuous new challenges. The half-life of skills is decreasing, with nine out of 10 UK workers expected to retrain by 2030. Our current education system structure provides a single touchpoint early in life, shaping how we will work and live for the subsequent decades.
Current education systems are also limiting human potential through generic prescriptive curricula that fail to take every learner’s needs into account. There is a chance to tap into the unique potential of all learners and equip them with life-centric skills. We need a shared vision for the role of education and teaching that puts human intelligence at its heart, while exploring the potential benefits artificial intelligence could bring.
Education has other flaws as a system; there has been a steady increase in UK school exclusions and a decline in adult learners’ engagement with learning. Experiences of exclusion disproportionately affect learners with special educational needs, those who are in care, and/or those from certain ethnic minority groups. In correlation with wider systemic factors, such as economic insecurity and unintended consequences of policy-making, these experiences lead to severed relationships at all levels within the education system.
During the roundtable, the purpose of our education system was scrutinised in anticipation of a conversation about where AI solutions might prove additive to prepare us for an exponential change in the skills landscape. Questions that arose included: what is the long-term goal of education beyond a certificate of achievement or attaining a specific job? When asked about the state of the UK’s current education system, one educator in the room stated it was “fantastic for preparing people for the 20th century rather than the 21st century”; the system can negatively impact learners’ confidence through arbitrary testing, rather than catering to a variety of skills, needs and aptitudes. Are there other education models that would better unlock a growth mindset for learners over their lifetimes?
Pinball Kids: preventing school exclusions
Report
Laura Partridge,Fran Landreth Strong,Elinor Lobley,Danielle Mason
Personalised learning could help meet these challenges
With the rise of AI technologies, some learners are already adopting AI to reshape the contours of their learning and harness better educational outcomes. One of the most profound shifts it has engendered is in the sphere of personalised learning.
Personalised learning represents an instructional approach where pedagogy, curriculum and learning environments are placed into the hands of individual learners to enable them to meet their own diverse needs and interests. Personalised learning is often celebrated as a concept, yet its definition and effectiveness remain points of contention. During our roundtable discussion, participants questioned if the term 'personalisation' conveys the essence of education in practice and emphasised the need for consensus on its effectiveness as an educational method. Further, the concept of personalised learning is frequently conflated with differentiated and individualised learning.
Differentiated learning involves tailoring instruction to align with the varying needs and goals of individual students, all within the context of shared academic objectives. In contrast, individualised learning caters to the unique pace and learning requirements of students, employing a standard curriculum for everyone. Distinctively, personalised learning entrusts and empowers students to own their learning while also addressing their individual needs and interests. It is an evidence-based approach that tailors content to fit individual learners' abilities, needs and objectives, thereby optimising their learning experience.
Currently, most learning management systems focus on individualised, not personalised learning. When applied properly and meaningfully, AI can facilitate the delivery of true personalised learning experiences. It can:
- facilitate lifelong learning and timely interventional responses from educators.
- involve parents more in their children’s learning.
- empower educators with data to support students.
- either simplify problems or make those problems more complex based on the individual students’ needs.
In this context, AI for personalised learning could be delivered through:
- A personalised programme of study: AI suggests module courses and ordering based on prior learning, interests and career goals.
- An individual course: AI guides you through an individual course, showing more or less material based on your learning progress. This is usually considered adaptive learning.
- Tailored content: AI produces content within a course, tailored to your needs. This could be to support a disability, to recommend additional material or to provide very personalised feedback.

AI: a feasible solution for personalised learning
AI is still an emerging technology and the way it is used varies among different sectors; within education, most institutions are at the early stage of AI application and maturity. The global AI in education market was valued at USD 1.82bn in 2021 and is expected to witness significant growth, with a compound annual growth rate of 36 percent from 2022 to 2030.
In response to the market trend, the UK has laid out its vision to become a global leader in artificial intelligence through the National AI Strategy. The UK Government's position paper on generative AI in education highlights the technology's vast potential, offering benefits such as personalised learning materials, creative support for students, immersive learning experiences, and enhanced efficiency in educational content creation. These combined efforts pave the way for a transformative future in the education field.
We can consider a typology for AI in education and learning through the lens of the Three Horizons (3H) model. It’s important to note that the discussion around AI for personalised learning is not just focused on traditional educational institutions such as schools, but extends to lifelong learning provision. Using the 3H model helps to ground conversations around AI in the current landscape, emerging trends and disruptive futures.
Our landscape review suggests AI in education applications can be broadly categorised under a typology consisting of adaptation, automation, conversation and generation. With reference to the 3H model, each of them progressively reshapes our understanding and expectations of education. Below we describe each horizon with examples of relevant AI innovations in the education and learning sector.
Horizon 1 (short-term) is about the immediate application of AI to optimise the current skills and learning system. Automation, exhibited by systems like Gradescope, Graide, and Mobius by Mobius by DigitalEd, enhances efficiency by providing instant feedback and performance analytics. These AI-powered grading systems analyse student work, providing instant feedback to both students and teachers, and detailed analytics on student performance to identify areas where additional support may be required.
Horizon 2 (medium-term) is about peak trends that may either be short-lived, or that may facilitate the transition to a transformed skills and learning system in the third horizon. The roles of conversation and generation types of AI in education technology come to the forefront. AI conversational systems, such as Jill Watson at Georgia Tech, Squirrel AI, TutorBot, and ChatGPT, provide on-demand student support and administrative assistance. Generation typology, demonstrated by platforms like CogBooks, leverages AI to create personalised educational content.
In Horizon 3 (long-term), the goal is to disrupt and transform the skills and learning system. Here, the adaptation typology is applicable, with systems like CENTURY, Whizz Education, Knewton, and Dreambox providing personalised instruction based on a student's individual learning needs. These AI-powered adaptive learning systems deliver personalised instruction, feedback and assessment by analysing student data to identify strengths and weaknesses, adjusting the content, style and difficulty levels accordingly.
The frontier of AI in education extends beyond these typologies, potentially reinventing our understanding of human intelligence, the purpose of learning and pedagogy. This interplay between the 3H model and AI typologies offers a rich narrative, tracing the evolution from personalised learning's current status to its potentially transformative future. It anchors our efforts in a forward-looking vision, where embracing current disruptions cultivates more equitable education outcomes in the future.
Perspectives from the roundtable participants spanned these core three horizons of AI for education to effectively speculate on both the changing education landscape and its requirements, and the potential of AI technologies to meet those demands.
AI and accelerated personalised lifelong learning
Could, would and should AI be applied to accelerate personalised lifelong learning? To begin interrogating these questions, we used three lenses and sub-questions to frame the discussion: the viability (would), desirability (should) and feasibility (could) of AI-enabled personalised learning.
Viability
- How might this ambition scale reach and personalisation so every learner achieves their best potential and what might hinder this?
- How might this ambition help or hinder equity and inclusion across learning and education opportunities?
- How might this ambition help or hinder longevity of impact that improves outcomes across education and learning for the generation to come?
Desirability
- How do current public and education sector behaviours and attitudes help or hinder this ambition, particularly in terms of adoption and promotion?
- How does the current public and education sector trust in AI and between industries and institutions help or hinder this ambition, particularly in relation to data governance, ownership and rigour?
- How does or might the approach to ethics and AI help or hinder this ambition in terms of societal values and undesirability. How do we ensure AI is used ethically and where should we try not to adopt AI?
Feasibility
- How does current AI-related technology advancements and capabilities help or hinder this ambition?
- How could policy and regulation create or hinder this ambition to grow and scale? And who should be responsible and accountable for ethical AI practice?
- How does financing and investment help or hinder this ambition to grow and scale?
There is a window of opportunity to integrate AI into current learning practices, but we have to consider carefully how this window is managed and who benefits. By assessing the present state of learning and its nascent relationship with AI with a diverse group of people with experience and expertise, we get to the heart of these questions. In part two, we use the three lenses of viability, desirability and feasibility to outline a set of potential opportunities for AI-enabled personalised learning to become a reality, alongside a set of prioritised barriers that are worth considering.
The illustrations used in this blog are lifted from Google DeepMind's Visualising AI collection. They are an artist's illustration of artificial intelligence and learning. They were created by Vincent Schwenk.

Cities of Learning
Connecting and catalysing place-based lifelong learning to unlock opportunities for a regenerative economy.
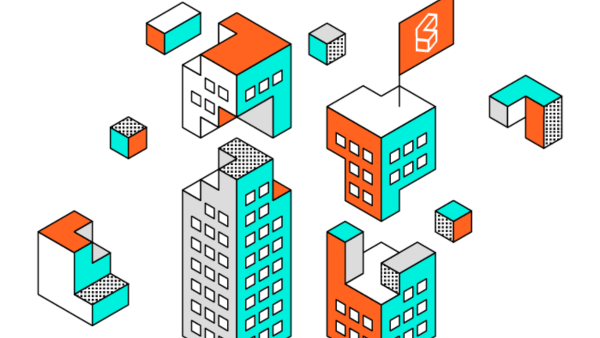
Join our virtual Cities of Learning network
Our dedicated Cities of Learning Circle community is full of place-based learning influencers. Interested? Get involved and help us develop the Cities of Learning movement with other Fellows online.
Read the other blogs in our AI and the future of learning series with Google DeepMind
-
AI-enabled personalised learning: opportunities and challenges
Blog
Alessandra Tombazzi Joanna Choukeir Natalie Lai DeepMind Partnership Block
In blog two of three in our series with Google DeepMind, we explore the opportunities for AI-enabled personalised learning as well as the projected barriers to achieving this future vision.
-
Future trajectories for AI-enabled personalised learning
Blog
Alessandra Tombazzi Joanna Choukeir Natalie Lai DeepMind Partnership Block
In the final article of three drawing insights from a multidisciplinary roundtable discussion on AI and learning we speculate on future scenarios and identify the next steps to enable AI for lifelong learning.
Be the first to write a comment
Comments
Please login to post a comment or reply
Don't have an account? Click here to register.